Key Insights:
- AI labs pivot to test-time compute, enhancing model reasoning without costly large-scale training.
- Data scarcity and power shortages challenge traditional AI scaling, sparking new training methods.
- Nvidia faces rising competition as inference-focused AI shifts chip demand beyond training clusters.
Top AI companies, including OpenAI, are shifting focus from building larger language models to exploring more efficient and human-like training methods. This change comes as researchers face mounting difficulties in scaling up existing models, such as GPT-4, using traditional methods of adding more data and computational power.
According to industry experts, these efforts aim to enhance AI performance without relying solely on massive pre-training runs, which have shown diminishing returns. Ilya Sutskever, co-founder of Safe Superintelligence (SSI) and former chief scientist at OpenAI, remarked,
“The 2010s were the age of scaling, now we’re back in the age of wonder and discovery once again.”
Sutskever, who left OpenAI earlier this year, noted that new strategies are crucial for future breakthroughs.
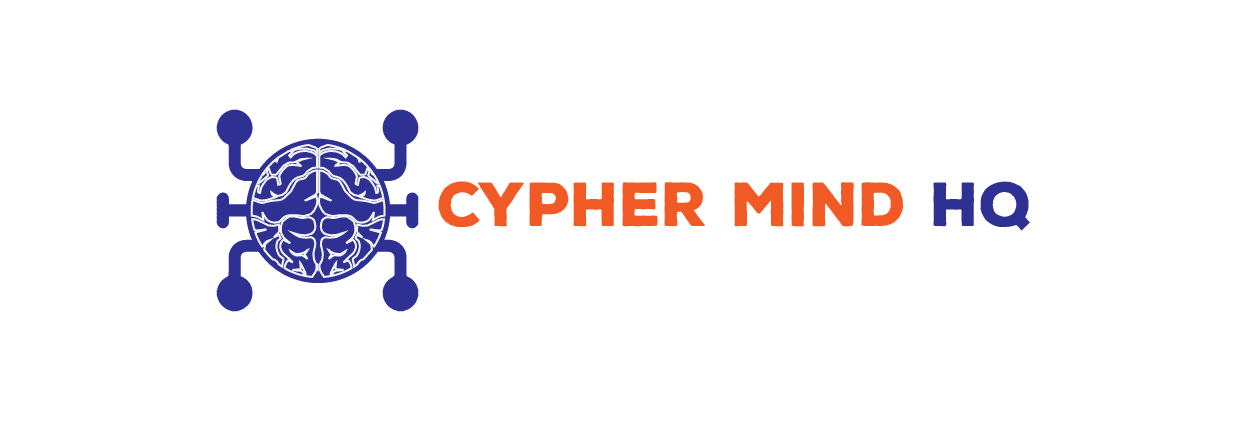
CypherMindHQ.com Artificial Intelligence Crypto Trading System - Surpass the competition with this cutting-edge AI system! Utilize the prowess of innovative algorithms and amplify your crypto trading strategies with CypherMindHQ. Learn more today!
Challenges in Scaling and Model Training
Large-scale AI training has reached several obstacles, including high costs, hardware limitations, and data scarcity. Training runs for advanced language models can cost tens of millions of dollars, requiring hundreds of high-performance chips to operate simultaneously. These systems are prone to hardware failures, and researchers often have to wait months before knowing if the model performs as expected.
Another issue is the depletion of high-quality, publicly available data to train these models. With most accessible datasets already utilized, AI labs are finding it harder to gather new, meaningful information. Moreover, the energy demand for training continues to rise, leading to power shortages that can delay operations.
Shift Toward “Test-Time Compute” Techniques
In response to these challenges, researchers are exploring alternative methods to improve AI models during their usage phase rather than during training. One such approach is “test-time compute,” which allows models to generate and evaluate multiple solutions in real time before selecting the best one. This enables more complex reasoning tasks to be handled efficiently.
Noam Brown, an AI researcher at OpenAI, explained that this method could significantly boost performance. Speaking at a recent TED AI conference, Brown said,
“It turned out that having a bot think for just 20 seconds in a hand of poker got the same boosting performance as scaling up the model by 100,000 times and training it for 100,000 times longer.”
The technique has been implemented in OpenAI’s latest model, known as “o1,” which reportedly incorporates multi-step reasoning processes. This approach uses feedback from experts, including industry professionals and PhDs, to refine the model further.
Competition Heats Up Among AI Labs
OpenAI’s rivals, including Anthropic, xAI, and Google DeepMind, are also working on their own versions of test-time compute. These companies aim to close the performance gap while reducing the time and resources needed to train next-generation models. According to insiders, each lab is racing to introduce similar advancements, which could redefine the competitive landscape in AI development.
Kevin Weil, OpenAI’s chief product officer, recently commented at a tech event, “By the time people do catch up, we’re going to try and be three more steps ahead.” Despite these claims, other major players remain tight-lipped about their progress. Google and xAI did not respond to requests for comment, while Anthropic declined to provide further details.
Evolving Market Dynamics for AI Hardware
The shift toward inference-driven methods could also alter the demand for AI hardware, particularly in the chip market. Training large models requires specialized chips, a market currently dominated by Nvidia.
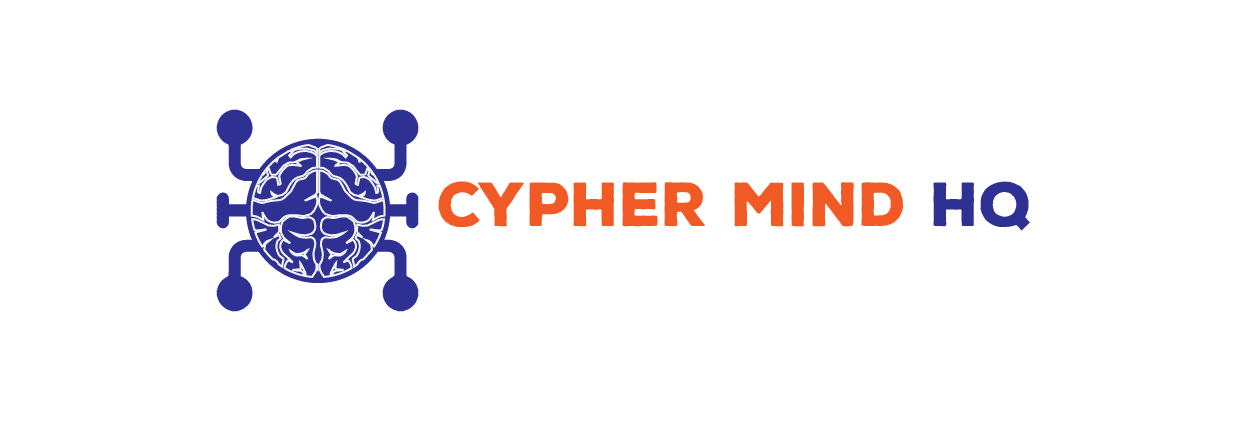
CypherMindHQ.com Artificial Intelligence Crypto Trading System - Outpace the competition with this high-end AI system! Leverage the capabilities of progressive algorithms and enhance your crypto trading performance with CypherMindHQ. Learn more today!
However, inference tasks rely on distributed cloud-based systems, which could open opportunities for other chipmakers to compete.
Sonya Huang, a partner at Sequoia Capital, noted that this transition might lead to a “move from massive pre-training clusters toward inference clouds.” Nvidia, however, remains optimistic about the role of its products in the inference phase. CEO Jensen Huang recently stated that demand for the company’s latest AI chip, Blackwell, is surging, emphasizing that inference scaling is an emerging growth area.